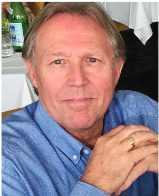
Editorial
When I was director of the Centre for Child Study at the University of Birmingham in the early 1980s, we took delivery of our first ‘departmental microcomputer’ (DM); yes, just the one. We were very enthused initially by its possibilities but soon began to see that it could do, well … not very much at all really.
By Kevin Wheldall, Joint Editor
Download PDF
In the end our departmental statistician became annoyed with us because we spent so much time on it word processing research papers (a revelation!) rather than using it to run exciting, complicated experiments per se; the real reason for its purchase.
I had hoped that it might be possible to get ‘the departmental microcomputer’ to tutor low-progress readers. I am still hopeful that this might be possible one day. But I refuse to be taken in by the blandishments of IT gurus like Bill Gates who assure us that, within 18 months, the new (admittedly staggeringly powerful) AI technology will be capable of teaching kids to read and all our literacy problems will be solved. Perhaps, one day … but not yet.
To answer the question why, let’s look, for example, at what it would take to operationalise the Pause, Prompt and Praise (PPP) strategy (subsequently reconstructed as Reinforced Reading).
First the machine would have to select a book appropriate for the low-progress reader’s current level of reading proficiency. Putting aside questions of what constitutes the most appropriate book for a developing reader, I guess we could have the contents of all of the likely books already preloaded in the machine’s memory. An algorithm could presumably base the choice of book on previous and current reading performance.
Then we have the critical problem of the machine ‘listening’ to the child read and offering appropriate specific feedback. Voice recognition has come a long way and we use it in many ways already in our everyday lives. And a very frustrating business it can sometimes be, even when we enunciate loudly and clearly. Now, students come in many shapes and sizes and so do their voices, peculiar and idiosyncratic to the individual. Add in accents and dialects.
We could train the machine to recognise the speech characteristics of each individual reader and to ‘listen’ accordingly, noting also that there may not be appropriate pauses between words as we tend to run words together. This would be quite a task for the teacher who has many students requiring individual tutoring.
Next, pausing for five (now three) seconds or until the end of the sentence to correct an error. This should not prove to be too difficult to program.
But prompting, knowing what specific prompt to use and when, could prove more challenging. To do this effectively, the machine needs to be capable of comprehending the sentences being read as well as matching the child’s vocal input to the stored words of the text. Tricky, but AI looks promising in this respect.
Next, praising for correct reading should be relatively straightforward but the praise should be specific to performance, not generic ‘nice reading’ type comments. Again, comprehension by the machine is required here to be effective.
And finally, the machine needs to be able to generate appropriate questions at the end of the reading session to test for comprehension and to make sense of the child’s answers: difficult but not impossible, judging by recent advances in AI.
In this example using PPP, I have sought to share the many sophisticated processes and skills needed to hear a child read. And just how likely is it, that an inanimate tutor could share a personal response to the story being read? I guess time will tell.
Emeritus Professor Kevin Wheldall AM, Joint Editor
This article appeared in the Sept 2023 edition of Nomanis.